Descriptive vs Inferential Statistics: Key Differences
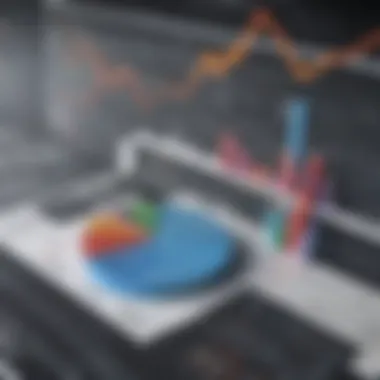
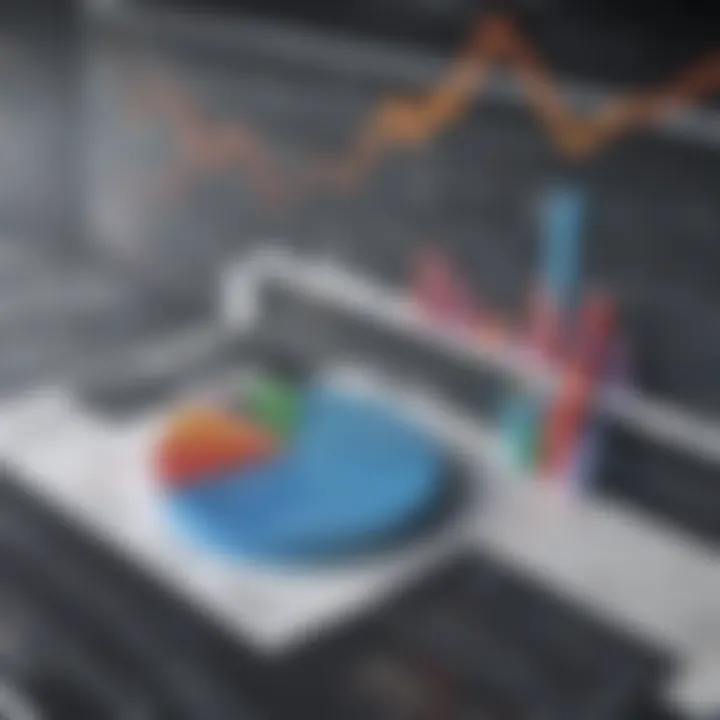
Intro
Understanding statistics is crucial in today's data-driven world. It not only informs decision-making but helps one grasp the nuances of information presented in various forms. At the heart of statistical analysis lies two significant branches: descriptive statistics and inferential statistics. Both serve distinct purposes yet intertwine to offer a comprehensive perspective on data.
Descriptive statistics focuses on summarizing and organizing data into an accessible format, shedding light on trends and patterns. On the flip side, inferential statistics takes a step further by using sample data to make broader conclusions about populations.
As we delve deeper into each section, the interplay between these two areas will reveal how they bolster data understanding and decision-making across various fields. Insights into their methodologies, applications, and significance will become clearer, helping readers navigate through the complexities of statistical analysis.
Understanding Statistics
Understanding statistics is crucial for anyone navigating the sea of data we face today. In research, statistics serve as the backbone, guiding us through analysis and interpretation of data. Without a clear grasp of statistics, one might find themselves lost, trying to decipher numbers without context or meaning. This article dives into the fundamental aspects of statistics, focusing on their significance and applications in various fields. It's not just about crunching the numbers; it's about extracting insights that can influence decision-making.
The Role of Statistics in Research
Statistics play a multifaceted role in research. Primarily, they aid in summarizing vast sets of data, making the information more digestible. Statistics can transform raw numbers into meaningful findings, helping researchers test theories and validate hypotheses. They also equip researchers with tools to handle uncertainty, which is inherent in any study. By employing statistical methods, researchers can convey how robust their findings are, allowing others to build upon or question their work.
Moreover, engaging with statistics fosters critical thinking. Researchers who understand statistics can question methodologies, scrutinize results, and interpret them accurately—not just at face value but within a broader context. This ability to assess the quality of data and its subsequent interpretation can dramatically affect the conclusions drawn and, ultimately, the practicality of research outcomes.
Types of Statistics
The world of statistics branches into two main categories, each with distinct purposes and characteristics: descriptive and inferential statistics. Knowing the difference is key for applying the right methodology in analysis.
Descriptive Statistics
Descriptive statistics serve to provide a comprehensive overview of data. They summarize and present quantitative descriptions in a manageable form. This could include calculating averages or displaying data in graphical formats, such as bar charts or histograms. The primary characteristic of descriptive statistics is its ability to depict the general features of a dataset without making inferences or predictions.
The unique feature of descriptive statistics is its straightforwardness and clarity. By presenting data summaries, it makes the job of understanding a dataset much simpler. However, relying solely on descriptive statistics can sometimes lead to oversimplicity. While they offer clarity, they do not account for randomness or variation within samples. This limitation makes them less effective when generalizing findings to larger populations.
Inferential Statistics
Conversely, inferential statistics provide the means to draw conclusions about populations based on sample data. This branch introduces concepts like hypothesis testing and regression analysis, which allow researchers to make predictions based on observed data. The core characteristic of inferential statistics lies in its power to generalize and infer, forming a bridge between a sample and the larger population from which it is drawn.
The unique advantage of inferential statistics is their potential to account for uncertainty and variability, offering deeper insights into data. However, they come with their own set of challenges. Misinterpretation of outcomes or misuse of statistical methods can lead to incorrect conclusions, which could have serious implications, especially in fields like market research or clinical trials.
"Statistics is the science of decision-making under uncertainty." This underscores the importance of choosing the right statistical approach.
Descriptive Statistics
Descriptive statistics plays a vital role in data analysis by summarizing and organizing the characteristics of a dataset. It’s like giving readers a quick snapshot or a concise overview of what the data looks like, allowing for easier understanding and interpretation. Particularly for investors, financial advisors, and students, having a clear grasp of descriptive statistics is not just beneficial; it can be the difference between making well-informed decisions or getting lost in a sea of numbers.
In this article section, we will delve into the definitions, purposes, key measures, and applications that define descriptive statistics and its overarching significance. Understanding these key components can empower individuals to utilize data more effectively in their decision-making processes.
Definition and Purpose
Descriptive statistics can be defined as the branch of statistics that focuses on collecting, summarizing, and presenting data in an informative way. Unlike inferential statistics, which aims to draw conclusions about a population based on a sample, descriptive statistics stays grounded within the data at hand. This distinctiveness allows for a direct insight into the dataset's properties, fostering a better understanding of underlying patterns or trends.
The purpose of descriptive statistics is manifold. Primarily, it helps to:
- Summarize large amounts of data into comprehensible metrics.
- Identify patterns or variations within the dataset.
- Aid in effective communication of findings to stakeholders.
Key Measures
Measures of Central Tendency
Measures of central tendency serve as techniques used to determine the center of a dataset. They include mean, median, and mode, each offering differing insights into the data's distribution.
Key Characteristics: The mean gives the average value, the median reflects the middle point, while the mode indicates the most frequently occurring data point.
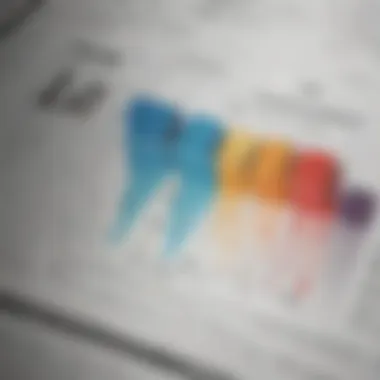
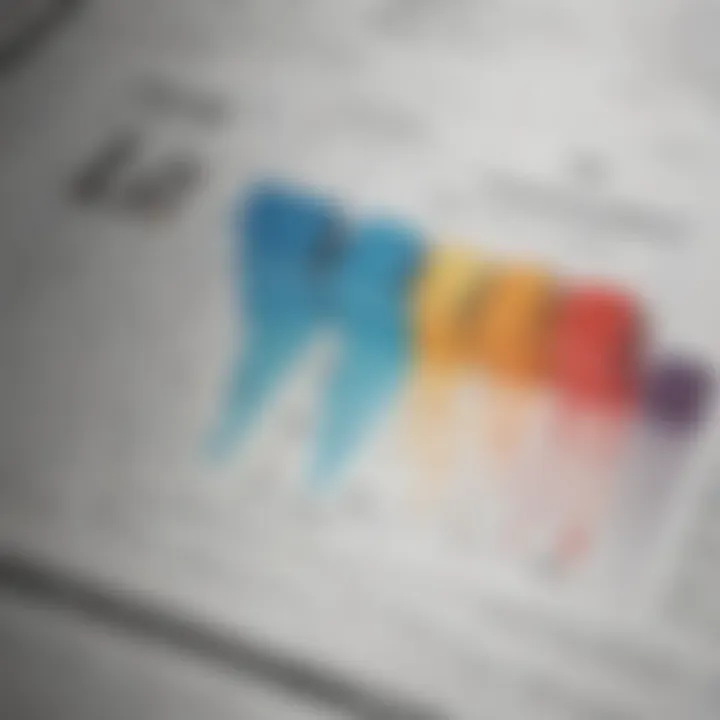
Significance: This diversity in measures is critical, as it allows one to choose the most suitable method based on the dataset’s characteristics. For instance, skewed distributions benefit from the median more than the mean.
Unique Features: A distinctive aspect of the measures of central tendency is their ability to condense extensive data into a single value, enhancing clarity. However, one should be cautious; they can sometimes mask diverse underlying distributions.
Measures of Variability
Measures of variability quantify how far the data deviate from the center. Common examples include range, variance, and standard deviation.
Key Characteristics: These measures help to compute the spread of data points in relation to the mean. The standard deviation, for instance, indicates how data points typically differ from the average.
Importance: Thus, descriptive statistics gains a richer dimension by identifying not just where data sits, but also how consistent or diverse it is.
Unique Features: One downside to measures of variability could be their complexity. For less experienced individuals, calculating standard deviation can seemingly add a layer of confusion.
Graphical Representation
Graphical representation involves utilizing visual formats—such as bar charts, pie charts, and histograms—to depict data points effectively.
Key Characteristics: This method allows audiences to visually grasp information at a glance. A well-designed chart can communicate complex concepts with immediacy.
Beneficial Nature: This aspect makes graphical representation a popular choice as it simplifies the communication of findings, persuading stakeholders with compelling visuals.
Unique Features: However, there's a caveat; poorly designed visuals can lead to misunderstandings or misinterpretations. Just like a double-edged sword, clarity in graphical representations is paramount.
Applications of Descriptive Statistics
Business Analytics
In the realm of business analytics, descriptive statistics supports companies by summarizing sales data, customer trends, or operational metrics.
Key Characteristics: It enables decision-makers to quickly analyze performance indicators.
Importance: The clarity that descriptive statistics brings is crucial for identifying areas in need of improvement or regions of strength.
Unique Features: While it effectively informs on current operations, it sometimes lacks predictive capability, leaving a gap that inferential statistics must fill.
Public Health
Descriptive statistics plays a significant role in public health by summarizing health-related data such as disease incidences or vaccination rates.
Key Characteristics: By consolidating complex information, public health officials can identify issues and allocate resources efficiently.
Importance: The direct insight derived from descriptive data means quicker responses to health crises, promoting better health outcomes.
Unique Features: Nevertheless, descriptive statistics can lead to oversimplification of complex health scenarios, making it important to rely on a combination of statistical methods for comprehensive analysis.
Education Assessment
In education assessment, descriptive statistics aids in evaluating student performance across various metrics, from exam scores to attendance rates.
Key Characteristics: This data allows educators and institutions to pinpoint areas needing enhancement, thus facilitating data-driven decision-making.
Importance: By presenting student data clearly, institutions can implement targeted programs for improvement.
Unique Features: However, these assessments could sometimes overlook qualitative aspects of education, necessitating a mixed-method approach to truly understand student experiences.
"Statistics are the quiet executors of decision-making processes; without them, informed choices are just conjectures."
Inferential Statistics
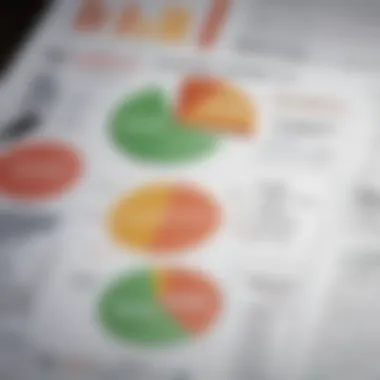
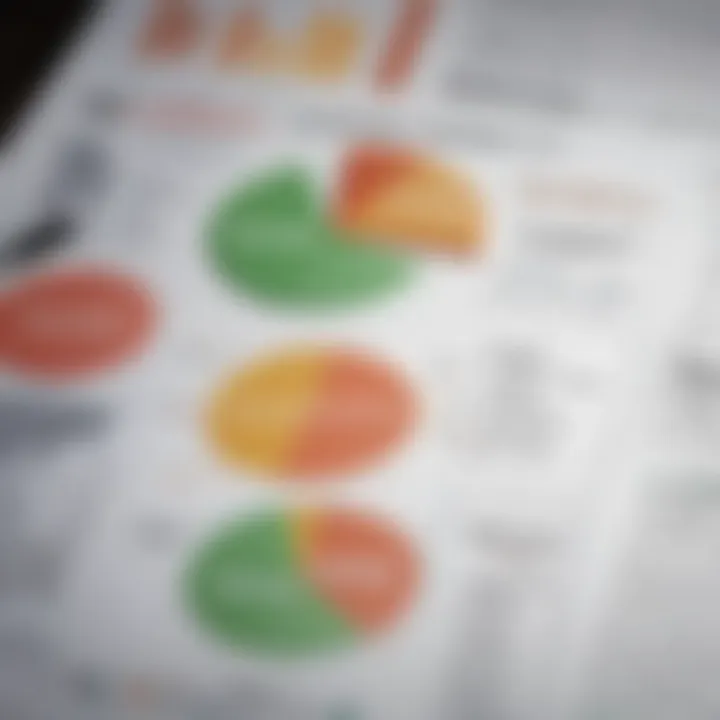
Inferential statistics plays a pivotal role in advancing our understanding of data analysis, illuminating pathways that descriptive data can only hint at. Its core lies in making predictions or generalizations about a larger population based on observations from a smaller sample. This leap from sample to population is not just a statistical exercise; it’s fundamental for informed decision-making across diverse fields such as market research, public health, and social sciences.
Definition and Purpose
Inferential statistics is not merely about crunching numbers; it’s about drawing the big picture from a snapshot. It provides methodologies that allow researchers to extrapolate findings from a sample to a population, enabling insights that can impact policies, business strategies, and scientific understanding. Without this branch of statistics, we would be looking at individual data points in isolation, missing the forest for the trees.
Fundamental Concepts
Population vs. Sample
The distinction between population and sample is a cornerstone of inferential statistics. A population encompasses every member of a group – think of it as the entire pie. In contrast, a sample represents a slice of that pie. Not always an easy slice to choose, it’s fundamental due to practical considerations; investigating an entire population can be time-consuming and resource-intensive.
A key characteristic of using samples is the potential for generalization. When researchers select a sample carefully, they can validly infer conclusions about the broader population. However, the unpredictability of sampling error may yield inaccurate representations, which is a consideration that must weigh heavily on researchers’ minds.
Sampling Techniques
Sampling techniques are the lifeblood of inferential statistics, acting as the bridge between a small dataset and broader conclusions. The elegance lies in how one selects units from a population. There are various methods—from random sampling that gives equal odds to each member, to stratified sampling, where the population is divided into subgroups before sampling.
The strength of these techniques is in their ability to enhance the reliability of results. However, the wrong sampling strategy can skew results and lead to false inferences, bringing us back to the drawing board.
Common Methods in Inferential Statistics
Hypothesis Testing
Hypothesis testing is like being a detective; it allows one to evaluate theories by testing propositions against observed data. This method helps to either accept or reject a proposed hypothesis. The critical aspect is setting a significance level—commonly at 0.05—which determines whether an outcome is rare enough to be considered significant.
This method is popular within the research realm due to its objective framework, though it comes with pitfalls. Misinterpretation can lead to erroneous conclusions, highlighting the need for careful, critical evaluation.
Confidence Intervals
Confidence intervals are another vital tool in the inferential statistics toolbox. They provide a range of values that likely includes the population parameter. For instance, if a data analysis offers a confidence interval of 40% to 60%, researchers can be relatively assured that the true value lies within that range, with a pre-defined level of confidence.
The distinct feature here is providing not just an estimate, but the uncertainty associated with that estimate. This approach is beneficial, yet it risks misleading if not communicated properly.
Regression Analysis
Regression analysis, featuring prominently within inferential statistics, serves to assess relationships between variables. Think of it as mapping the connection between advertising spend and sales figures. Researchers can discern how changes in one variable affect another, providing tremendous insight into trends and forecasting.
This method of scrutinizing relationships is invaluable. However, one must be cautious of overestimating the strength of correlations without considering confounding variables that may distort interpretations.
Applications of Inferential Statistics
Market Research
In market research, inferential statistics is corner-stone to understanding consumer behavior. For example, surveys conducted on a sample group can influence major business decisions, from product launch strategies to customer service improvements. By interpreting sample data, brands can predict customer needs more accurately—an invaluable edge in a competitive market.
However, businesses must be vigilant; rely on poor sampling methods, and the entire strategy becomes suspect.
Clinical Trials
Clinical trials utilize inferential statistics to evaluate the effectiveness of treatments. A diverse sample representing the larger population provides insights that challenge or reinforce medical theories. It’s a balancing act of ethical considerations and the need for statistically sound results.
While trials can yield significant advancements in healthcare, misinterpretations can lead to adverse outcomes, emphasizing the need for rigorous adherence to proper methodologies.
Social Science Studies
In social sciences, understanding and interpreting human behavior relies heavily on inferential statistics. For instance, using surveys to grasp public opinion on policies can shape political campaigns and societal decisions. Researchers can apply these insights to inform discussions on social change or policy reform based on solid data interpretation.
Yet, like other fields, the imperative remains: results must be speaker to context and validated carefully to ensure that inferences drawn are not merely artifacts of sampled data.
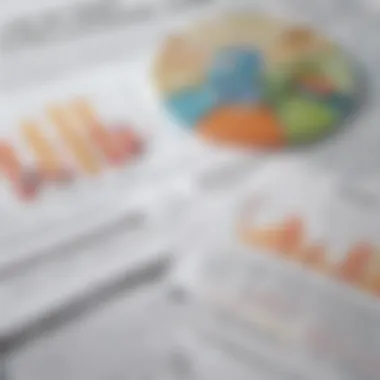
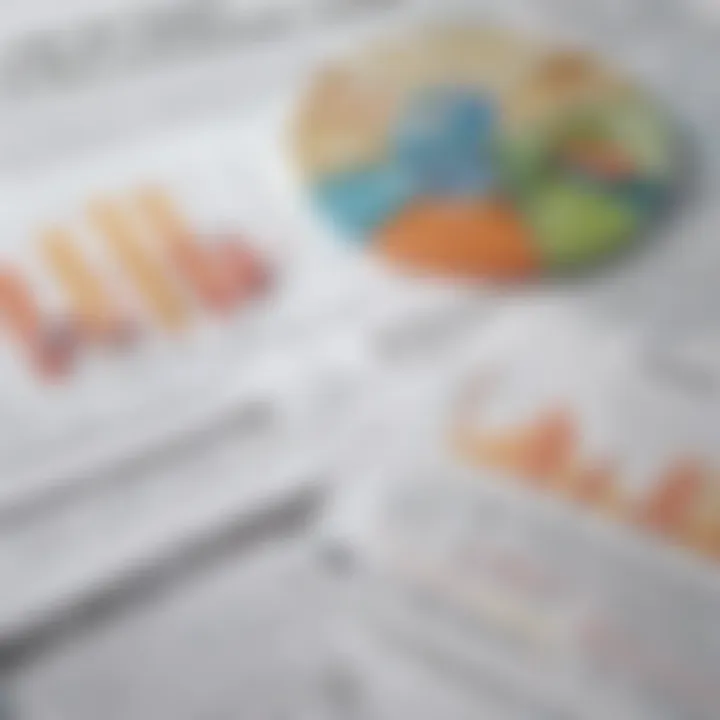
The strength of inferential statistics lies not only in its methodologies but also in its capacity to inform and shape decisions across myriad sectors, translating samples into significant insights that can guide both businesses and researchers alike.
Comparative Analysis
A comparative analysis serves as a crucial bridge between two distinct yet interconnected realms of statistics: descriptive and inferential statistics. Understanding how these two domains differ and where they overlap is foundational for anyone engaged in statistical evaluation. Each branch of statistics provides a unique perspective and brings its own strengths to the table.
It is essential to recognize the various aspects of data handling, interpretation, and decision-making that these two approaches define. By contrasting them, one can gain a richer understanding of statistical application in practical scenarios, which is especially relevant for investors, financial advisors, and students who depend on accurate data analysis for informed choices.
Descriptive vs. Inferential Statistics
Nature of Data
The nature of data in this context mainly refers to how information is collected, categorized, and analyzed. Descriptive statistics predominantly handle raw data, focusing on summarization and representation without venturing beyond the observed values. This analysis is beneficial as it offers a snapshot of the dataset at hand, making it easier for one to interpret the collected information.
In contrast, inferential statistics shifts the spotlight to samples drawn from a larger population, which imbues it with an inherent uncertainty. A key characteristic of inferential statistics is that it aims to extrapolate findings based on limited observations. This can be incredibly powerful for drawing conclusions, though it comes with risks, especially if the sampling isn’t representative. The unique feature here is the balance between insight and speculation—offering predictions while relying on assumptions, making it both a versatile and challenging approach in statistical analysis.
Goals of Analysis
The goals of analysis underpin the very reason for undertaking descriptive or inferential statistics. Descriptive statistics strive primarily to summarize vast datasets in clear, comprehensible formats. This has a direct impact on enabling businesses to visualize trends and deviations at a glance. As such, this method garners favor among those who seek clarity over uncertainty.
On the other hand, the aims of inferential statistics resonate deeply with those venturing into predictive analytics. It seeks to not only understand the current state but also to anticipate future occurrences based on the information gleaned from samples. This goal makes it a favorite among researchers who wish to test hypotheses or determine relationship dynamics within data. However, the unique feature of this approach—its potential to mislead if underlying assumptions aren’t met—highlights the necessity for caution when drawing conclusions.
Consequences of Misinterpretation
Misinterpretation of statistical data can have serious ramifications. When descriptive statistics are misunderstood, it can lead to oversimplified conclusions, painting an incomplete picture of reality. For instance, a company might decide to forgo an investment opportunity because its short-term performance appears unfavorable, without realizing long-term potential shown in broader trends.
Conversely, the risks associated with misinterpretation in inferential statistics are often more profound. Incorrect assumptions about a sample can misguide critical business or research decisions. For example, relying on flawed hypothesis tests might lead to unwarranted product launches or regulatory actions. It’s imperative for professionals and students alike to approach their analyses with a discerning eye, carefully considering their methods and the context behind their data.
In summary, the comparative analysis of descriptive and inferential statistics sheds light on how both branches serve distinct yet complementary purposes in statistical analysis. Each comes with its own advantages and challenges, making a deep understanding of both crucial for sound decision-making and effective data interpretation.
The Importance of Context in Statistical Analysis
When diving into the world of statistics, it’s all too easy to get lost in the quantitative labyrinth, crunching numbers without grasping the environment in which they exist. Context plays a pivotal role in shaping the interpretation of statistical findings. Without it, data can be manipulated, misinterpreted, and seriously misrepresented. The data collected could suggest one thing statistically, yet hold an entirely different meaning in the real world.
Understanding context includes recognizing the cultural, temporal, and situational variables surrounding the data. For instance, economic data from one region may have little relevance in another if cultural attitudes towards spending differ significantly. Here, considering these nuances can change how the statistics are perceived and implemented in decision-making processes.
Choosing the Right Approach
Selecting between descriptive and inferential statistics heavily depends on the context of the data and the questions being posed.
Descriptive statistics are handy when the intent is to paint a clear picture of what the data shows on its own, summarizing key characteristics such as mean, median, and mode in a manageable manner. If a business is analyzing sales performance for a particular quarter, descriptive data, like average sales per product, provides direct insight into that timeframe.
On the flip side, inferential statistics take a broader lens. If you’re interested in forecasting future sales based on the quarter's performance, then inferential methods come into play. These methods rely on sample data to make predictions or draw conclusions about larger populations, and here, the context, like market trends and consumer behaviors, needs proper evaluation to ensure reliability and accuracy.
Ethical Considerations
The ethical implications of statistical analysis cannot be overlooked. Misrepresentation of data can lead to misguided policies, financial discrepancies, and even public unrest. For instance, consider how clinical trial results are often scrutinized. If companies report only the positive outcomes while burying negative side effects, it misconstrues the efficacy and safety of the treatments involved.
Being transparent about the statistical methods used and acknowledging limitations of the analysis are crucial for ethical practices. This transparency builds trust with stakeholders, whether they are investors, clients, or the general public. Too often, statistics can be used as a smoke and mirrors game; presenting them out of context can skew perspectives.
In light of this, the ethical responsibility lies in choosing the right methodologies, ensuring accurate reporting and understanding the audience receiving the data. The aim should always be to inform, not to mislead. Therefore, paying attention to context also enhances ethical statistical practice, ensuring decisions are supported by sound data grounded in reality.
In summary, recognizing the vital role of context within statistical analysis enables more accurate interpretations and fosters ethical handling of data.
Finale
As we draw to a close on this examination of descriptive and inferential statistics, it becomes evident how intertwined these branches of statistical analysis are. Understanding the conclusion derived from analyzing these methods can lead to better data-informed decisions.
Summary of Key Points
- Descriptive Statistics provides a foundation by summarizing raw data. It allows researchers to report findings clearly, turning heaps of numbers into meaningful insights. Its measures, such as averages and graphing techniques, strip away the noise and give us a clear picture of what's going on within the dataset.
- Inferential Statistics, on the other hand, builds on those summaries. By using samples, it makes predictions about broader populations, offering insights that can inform business strategies or public policy. Through hypothesis testing and confidence intervals, it extends the understanding gained from descriptive analysis into realms of future expectations and assumptions.
- The importance of context in applying these methods cannot be overstated. Utilize descriptive stats to review current situations, while inferential methods help us make educated guesswork about future possibilities. Relevant statistical methodologies are unavoidably tied to the specific questions being asked.
- Readers from varied backgrounds— investors, financial advisors, students—can draw critical insights from this synergy.
Future Directions in Statistical Research
Looking ahead, the landscape of statistical research may witness several shifts that could redefine both descriptive and inferential approaches.
- Integration with Technology: As machine learning and AI are starting to take a foothold, we might see more automated methods for analyzing data, which could combine both descriptive and inferential techniques seamlessly. Algorithms will likely interpret vast datasets faster than a human ever could, allowing for real-time insights.
- Enhanced Predictive Analytics: There’s a growing focus on how statistical analysis can transition into predicting future trends more accurately, particularly in fields like finance and healthcare. This trend emphasizes the importance of inferential statistics as we strive to understand not just the present but the future as well.
- Interdisciplinary Applications: The future may also involve merging statistics with fields such as psychology, economy, and social sciences, leading to innovative methodologies that enhance our comprehension of complex datasets.
To wrap up, both descriptive and inferential statistics pose significant potential in transforming data into actionable knowledge. By being informed and discerning in our application of these methods, we can wield statistics more powerfully in various domains.